“The only constant in life is change.”
Heraclitus
AI-enhanced workflow automation software has transformed how businesses operate, streamlining processes and driving efficiency. Yet, one pressing issue that remains a significant challenge for many organizations is scalability. As businesses grow and evolve, their workflow automation systems must be able to scale accordingly to handle increased demand and complexity. This article examines how AI-driven workflow automation software addresses scalability challenges, supported by comprehensive factual data, real-world applications, and an analysis of broader implications for businesses.
Understanding the Scalability Challenge
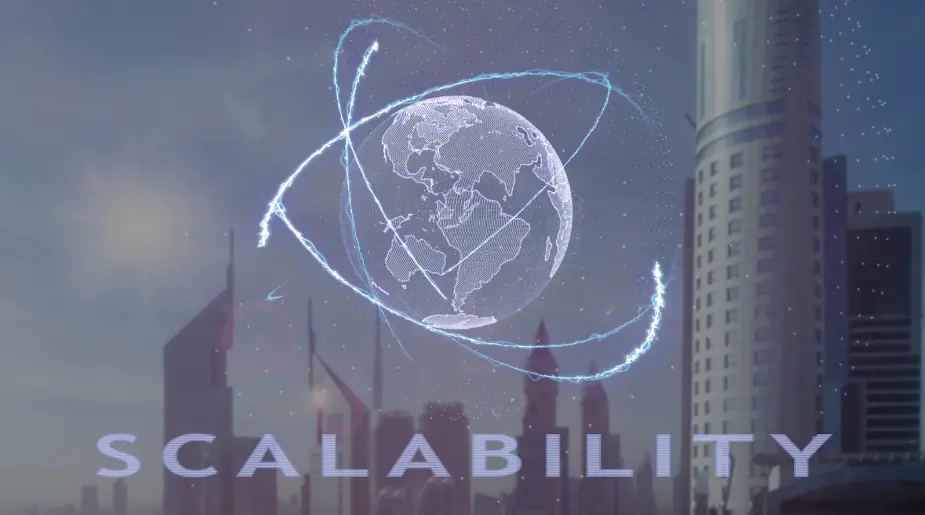
Scalability is a critical factor for the success of workflow automation systems. It refers to the ability of these systems to expand and adapt to increased workloads without compromising performance or efficiency. As organizations grow, they must process larger volumes of data, manage more complex workflows, and integrate additional systems and applications. However, many traditional workflow automation systems struggle to scale effectively, leading to performance bottlenecks, increased latency, and operational inefficiencies.
According to a report by McKinsey & Company, 45% of large enterprises cite scalability as one of their top challenges in adopting new technologies. This statistic underscores the importance of developing scalable solutions that can grow with the organization. The ability to scale seamlessly is crucial for maintaining the efficiency and reliability of automated workflows, especially in dynamic and fast-growing business environments.
AI Solutions for Enhancing Scalability
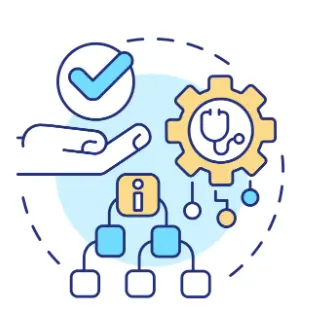
Artificial Intelligence offers robust solutions for enhancing the scalability of workflow automation systems. One key advancement is the use of AI-driven load balancing and resource optimization. AI algorithms can dynamically allocate resources based on real-time workload demands, ensuring that the system operates efficiently even as the workload increases. This approach minimizes performance bottlenecks and ensures that workflows run smoothly under varying conditions.
For example, AI can analyze historical data to predict peak usage times and adjust resource allocation accordingly. By optimizing the use of computational resources, AI-driven load balancing ensures that the system can handle increased workloads without compromising performance. A study by IDC highlights that AI-driven resource optimization can improve system efficiency by up to 40%, demonstrating the significant impact of AI on scalability.
Another significant application of AI in enhancing scalability is the use of distributed computing and cloud-based solutions. AI-driven workflow automation platforms can leverage distributed computing to process large volumes of data across multiple servers, ensuring that the system can scale horizontally. Cloud-based solutions provide additional flexibility by allowing organizations to scale their infrastructure on-demand, without the need for significant upfront investments in hardware.
AI also enhances scalability through advanced data processing techniques. AI algorithms can preprocess and compress data, reducing the volume of data that needs to be processed and stored. This approach minimizes the computational load and ensures that the system can handle larger datasets efficiently. According to a report by Deloitte, companies that leverage AI-driven data processing can reduce data storage costs by up to 30%, highlighting the efficiency gains achieved through AI-enhanced scalability.
Real-World Applications and Benefits
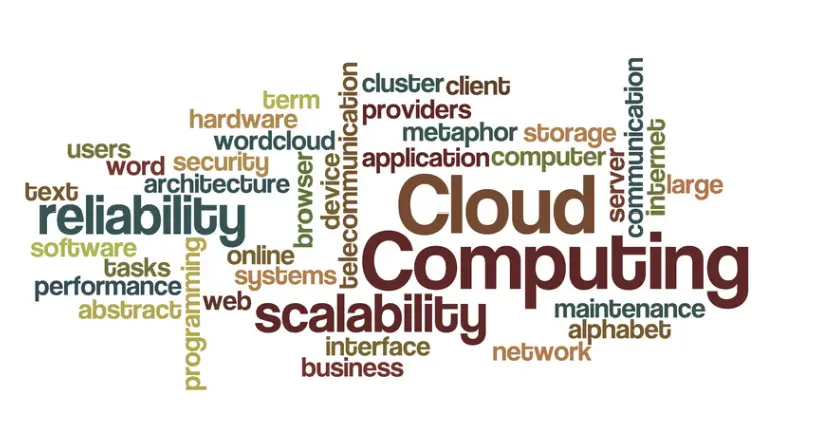
The practical application of AI in addressing scalability challenges is evident in various innovative workflow automation platforms. Companies like IBM and Microsoft have developed AI-powered solutions that prioritize scalability and performance.
IBM’s AI-driven automation platform, IBM Automation, uses advanced load balancing and resource optimization algorithms to ensure scalability. The platform dynamically allocates resources based on real-time workload demands, ensuring that workflows run smoothly even under heavy loads. IBM Automation also leverages distributed computing and cloud-based solutions to provide additional flexibility and scalability, allowing organizations to scale their infrastructure on-demand.
Microsoft’s Azure Logic Apps is another example of an AI-enhanced workflow automation platform that addresses scalability challenges. Azure Logic Apps uses AI-driven data processing techniques to preprocess and compress data, ensuring that the system can handle larger datasets efficiently. The platform also leverages cloud-based solutions to provide on-demand scalability, allowing organizations to expand their infrastructure as needed. These AI-driven capabilities ensure that Azure Logic Apps can scale seamlessly with the organization’s growth, maintaining efficiency and reliability.
Navigating the Challenges and Considerations
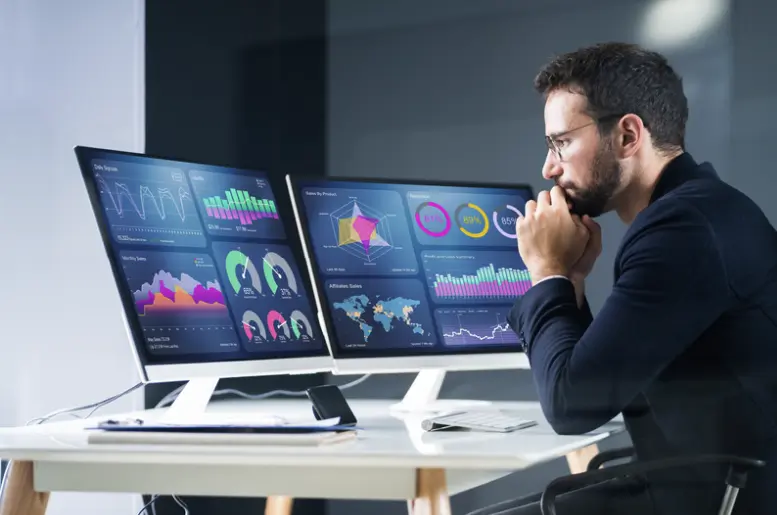
While AI offers significant benefits in enhancing scalability, there are several challenges and considerations to address. One primary concern is ensuring that AI-driven scalability solutions are reliable and secure. Organizations must ensure that their AI systems are robust and resilient to handle increased workloads without compromising security. Continuous monitoring and testing of AI algorithms are essential to maintaining reliability and performance.
Data privacy and security are also critical considerations. AI-driven scalability solutions process large volumes of sensitive data, making robust encryption and data protection measures essential. Transparency regarding data collection, storage, and usage policies is crucial to address privacy concerns and build confidence in AI-driven scalability solutions.
The cost of AI-enabled scalability solutions can also be a barrier to adoption. High-quality AI systems that provide advanced load balancing, distributed computing, and data processing capabilities can be expensive. Ensuring that these systems are affordable and accessible is crucial for broader adoption and enhanced scalability. Organizations must work together with technology providers to develop cost-effective solutions that do not compromise on quality and effectiveness.
Conclusion
AI-enhanced workflow automation software represents a significant advancement in addressing the challenge of scalability. By leveraging advanced technologies such as AI-driven load balancing, distributed computing, and data processing, AI can provide a comprehensive and efficient solution to ensure seamless scalability. These systems offer organizations unprecedented levels of flexibility, efficiency, and reliability, ensuring that automated workflows can scale with the organization’s growth.
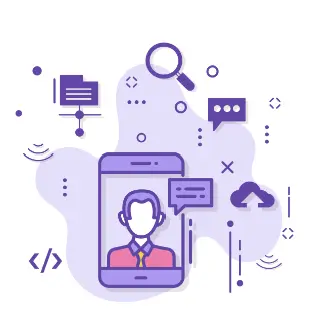
As technology continues to evolve, investing in AI-driven scalability solutions will become increasingly important for ensuring comprehensive workflow automation. Addressing challenges such as reliability, data privacy, and cost will be crucial to fully realizing the potential of AI in workflow automation. Ultimately, AI represents a transformative force in the realm of workflow automation, offering innovative solutions that enhance scalability, efficiency, and business outcomes.
For further insights into AI and scalability in workflow automation, refer to McKinsey & Company’s report on technology adoption and IDC’s study on AI-driven resource optimization.