“Data is the new oil.”
Clive Humby
The adoption of AI-enhanced workflow automation software has transformed business operations, enabling organizations to achieve unprecedented efficiency and productivity. However, a significant challenge that organizations face in implementing these systems is data integration. Effective workflow automation requires seamless integration of data from various sources, but disparate data formats, legacy systems, and data silos can complicate this process. This article examines how AI-enhanced workflow automation software is addressing data integration challenges, supported by comprehensive factual data, real-world applications, and an analysis of the broader implications for businesses.
The Complex Landscape of Data Integration
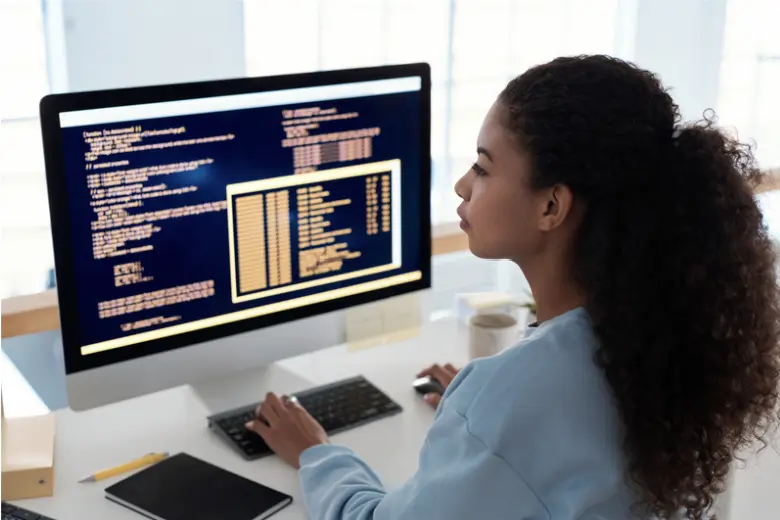
Data integration is a critical component of workflow automation, as it involves combining data from different sources to provide a unified view. This process is essential for ensuring that automated workflows can access and utilize relevant data across the organization. However, data integration can be complex due to several factors.
Firstly, organizations often use multiple systems and applications, each with its own data format and structure. This diversity can create challenges in merging data into a cohesive framework. For example, customer data might be stored in a CRM system, financial data in an ERP system, and operational data in various other applications. Integrating this data into a unified workflow requires significant effort and expertise.
Secondly, legacy systems pose a substantial hurdle. Many organizations rely on outdated systems that were not designed to integrate with modern technologies. These systems may use proprietary formats or lack the APIs necessary for data exchange, making integration difficult. According to a survey by Dell Boomi, 55% of organizations cite legacy systems as a major obstacle to data integration.
Additionally, data silos within organizations can impede effective data integration. Data silos occur when different departments or business units store data independently, without sharing it across the organization. This lack of data sharing can lead to inconsistencies and inefficiencies in automated workflows. A study by McKinsey & Company found that companies that effectively break down data silos can increase productivity by up to 20%.
AI-Driven Solutions for Data Integration
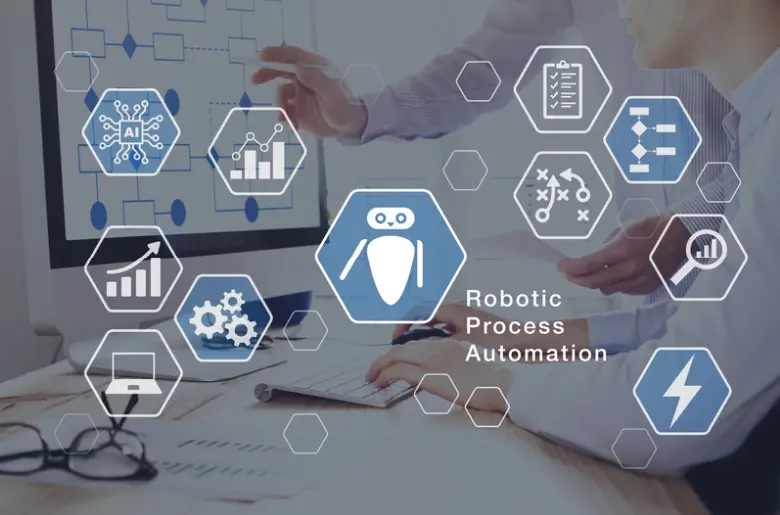
Artificial Intelligence offers powerful solutions to address data integration challenges in workflow automation. One of the key advancements is the use of AI-driven data mapping and transformation. AI algorithms can automatically map data from different sources and transform it into a consistent format. This capability simplifies the integration process and ensures that data is accurate and usable across workflows.
For instance, AI can analyze the structure and semantics of data from various systems and generate mapping rules that align the data with a unified schema. This process eliminates the need for manual data mapping, reducing the time and effort required for integration. A report by Accenture highlights that AI-driven data integration can accelerate the deployment of workflow automation solutions by 50%, demonstrating the efficiency gains achieved through automation.
Another significant application of AI in data integration is the use of natural language processing (NLP) to understand and interpret unstructured data. Many organizations have vast amounts of unstructured data, such as emails, documents, and social media posts, that need to be integrated into automated workflows. AI-powered NLP can extract relevant information from these unstructured sources and convert it into structured data, making it accessible for workflow automation.
AI also enhances data integration through machine learning-based anomaly detection. During the integration process, data inconsistencies or errors can occur, leading to inaccurate or incomplete workflows. AI algorithms can continuously monitor data integration processes and detect anomalies, such as missing values or inconsistent formats. By identifying and addressing these issues in real-time, AI ensures that integrated data is reliable and accurate.
Practical Applications and Benefits
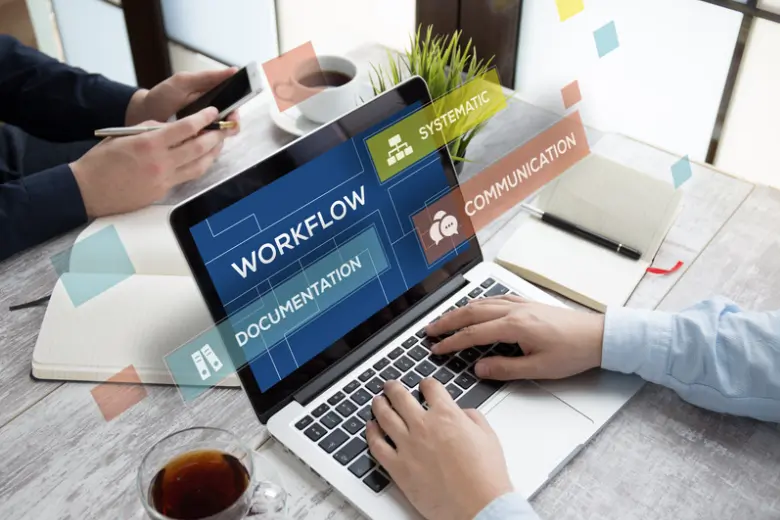
The practical application of AI in overcoming data integration challenges is evident in various innovative workflow automation platforms. Companies like MuleSoft and Dell Boomi have developed AI-powered solutions that streamline data integration and enhance workflow automation.
MuleSoft’s Anypoint Platform uses AI-driven data mapping and transformation to facilitate seamless integration across diverse systems. The platform’s AI algorithms automatically generate data mappings, reducing the complexity of integrating data from multiple sources. Additionally, MuleSoft’s Anypoint Platform leverages machine learning to continuously improve data integration processes, ensuring that workflows remain accurate and efficient as data sources evolve.
Dell Boomi’s integration platform also leverages AI to enhance data integration. Boomi’s AI-powered data mapping engine analyzes data from various systems and generates integration rules that align with a unified schema. The platform’s NLP capabilities enable the extraction of relevant information from unstructured data sources, making it accessible for workflow automation. Furthermore, Boomi’s machine learning algorithms monitor integration processes and detect anomalies, ensuring data integrity and reliability.
Navigating the Challenges and Considerations
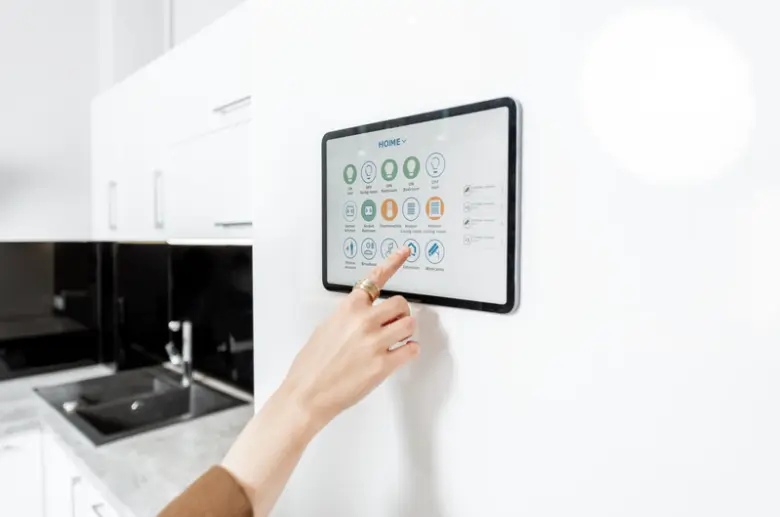
While AI offers significant benefits in enhancing data integration for workflow automation, there are several challenges and considerations to address. One primary concern is ensuring that AI-driven data integration solutions are compatible with a wide range of systems and applications. Organizations must ensure that their AI solutions can seamlessly integrate with existing and future technologies to achieve comprehensive data integration.
Data privacy and security are also critical considerations. AI-driven data integration involves processing large volumes of sensitive information, making robust encryption and data protection measures essential. Transparency regarding data collection, storage, and usage policies is crucial to address privacy concerns and build confidence in AI-driven integration solutions.
The cost of AI-enabled data integration solutions can also be a barrier to adoption. High-quality AI systems that provide advanced data mapping, transformation, and anomaly detection capabilities can be expensive. Ensuring that these systems are affordable and widely available is crucial for broader adoption and enhanced productivity. Organizations must work together with technology providers to develop cost-effective solutions that do not compromise on quality and effectiveness.
Conclusion
AI-enhanced workflow automation software represents a significant advancement in addressing the challenge of data integration. By leveraging advanced technologies such as AI-driven data mapping, natural language processing, and machine learning-based anomaly detection, AI can provide a comprehensive and efficient solution to ensure seamless data integration. These systems offer organizations unprecedented levels of accuracy, efficiency, and reliability, ensuring that automated workflows can access and utilize relevant data across the organization.
As technology continues to evolve, investing in AI-driven data integration solutions will become increasingly important for ensuring comprehensive workflow automation. Addressing challenges such as compatibility, data privacy, and cost will be crucial to fully realizing the potential of AI in workflow automation. Ultimately, AI represents a transformative force in the realm of workflow automation, offering innovative solutions that enhance data integration, productivity, and business outcomes.
For further insights into AI and data integration in workflow automation, refer to Accenture’s report on AI-driven data integration and McKinsey & Company’s study on breaking down data silos.