“Quality is not an act, it is a habit.”
Aristotle
The advent of AI-enhanced workflow automation software has revolutionized business operations, enabling unprecedented levels of efficiency and productivity. However, one critical challenge that organizations frequently encounter is ensuring data quality. The effectiveness of automated workflows hinges on the accuracy, consistency, and reliability of the underlying data. This article delves into how AI-driven workflow automation software addresses the issue of data quality, supported by comprehensive factual data, real-world applications, and an analysis of broader implications for businesses.
The Criticality of Data Quality in Workflow Automation
Data quality is a fundamental aspect that influences the success of workflow automation. Poor data quality can lead to incorrect decision-making, inefficiencies, and operational disruptions. According to a study by Gartner, poor data quality costs organizations an average of $15 million annually. This figure underscores the significant financial impact that data quality issues can have on businesses.
In the context of workflow automation, data quality encompasses various dimensions, including accuracy, completeness, consistency, and timeliness. Inaccurate or incomplete data can lead to flawed outputs and unreliable automation processes. Similarly, inconsistent data formats and delays in data availability can disrupt automated workflows, leading to inefficiencies and errors. Ensuring high-quality data is therefore crucial for the seamless operation of AI-enhanced workflow automation systems.
AI Solutions for Enhancing Data Quality
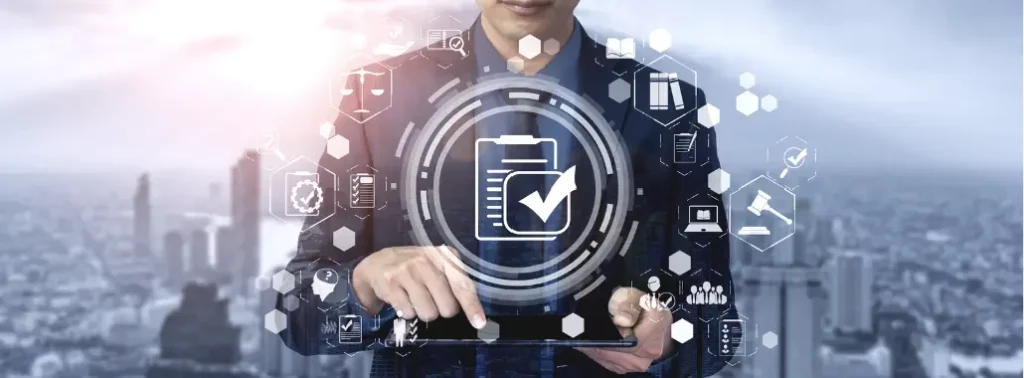
Artificial Intelligence offers powerful solutions for addressing data quality issues in workflow automation. One significant advancement is the use of AI-driven data cleansing and validation. AI algorithms can automatically detect and correct errors in data, ensuring that it is accurate and reliable. For example, AI can identify and rectify inconsistencies in data formats, fill in missing values, and correct typographical errors. This automated data cleansing process reduces the time and effort required for manual data correction, enhancing overall data quality.
AI also enhances data quality through advanced data integration techniques. AI-driven data integration tools can combine data from various sources, ensuring that it is consistent and harmonized. These tools use machine learning algorithms to identify and resolve data conflicts, ensuring that integrated data is accurate and reliable. According to a report by McKinsey & Company, companies that leverage AI for data integration can reduce data management costs by up to 30%, demonstrating the efficiency gains achieved through AI-enhanced data integration.
Another significant application of AI in enhancing data quality is the use of predictive analytics for data quality monitoring. AI algorithms can analyze historical data patterns and identify potential data quality issues before they impact workflow automation processes. For instance, AI can predict when data from certain sources is likely to be incomplete or inaccurate and flag these issues for further investigation. This proactive approach ensures that data quality issues are addressed promptly, minimizing their impact on automated workflows.
Practical Applications and Benefits
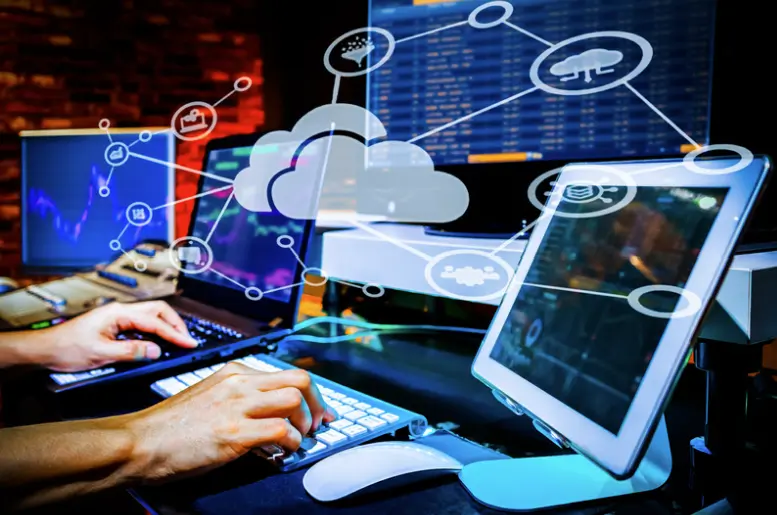
The practical application of AI in addressing data quality challenges is evident in various innovative workflow automation platforms. Companies like Talend and Informatica have developed AI-powered solutions that prioritize data quality and integration.
Talend’s Data Fabric platform uses AI-driven data cleansing and validation to ensure high-quality data for workflow automation. The platform’s AI algorithms automatically detect and correct errors in data, ensuring that it is accurate and reliable. Additionally, Talend’s AI-driven data integration tools harmonize data from various sources, ensuring that integrated data is consistent and reliable. Talend’s platform also leverages predictive analytics to monitor data quality, identifying potential issues before they impact workflow automation processes.
Informatica’s Intelligent Data Management Cloud is another example of AI-enhanced data quality solutions. Informatica’s platform uses AI-driven data cleansing and validation to ensure high-quality data. The platform’s AI algorithms also facilitate advanced data integration, ensuring that data from various sources is harmonized and consistent. Additionally, Informatica’s platform uses predictive analytics to monitor data quality, identifying potential issues and addressing them proactively. These AI-driven capabilities ensure that data quality is maintained, enhancing the effectiveness of workflow automation.
Navigating the Challenges and Considerations
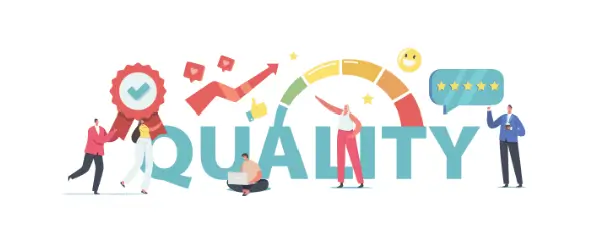
While AI offers significant benefits in enhancing data quality, there are several challenges and considerations to address. One primary concern is ensuring that AI-driven data quality solutions are accurate and reliable. Organizations must ensure that their AI systems are trained on comprehensive and up-to-date data to avoid errors and omissions. Continuous validation and testing of AI algorithms are essential to maintaining accuracy and reliability.
Data privacy and security are also critical considerations. AI-driven data quality solutions process large volumes of sensitive information, making robust encryption and data protection measures essential. Transparency regarding data collection, storage, and usage policies is crucial to address privacy concerns and build confidence in AI-driven data quality solutions.
The cost of AI-enabled data quality solutions can also be a barrier to adoption. High-quality AI systems that provide advanced data cleansing, validation, and predictive analytics capabilities can be expensive. Ensuring that these systems are affordable and accessible is crucial for broader adoption and enhanced data quality. Organizations must work together with technology providers to develop cost-effective solutions that do not compromise on quality and effectiveness.
Conclusion
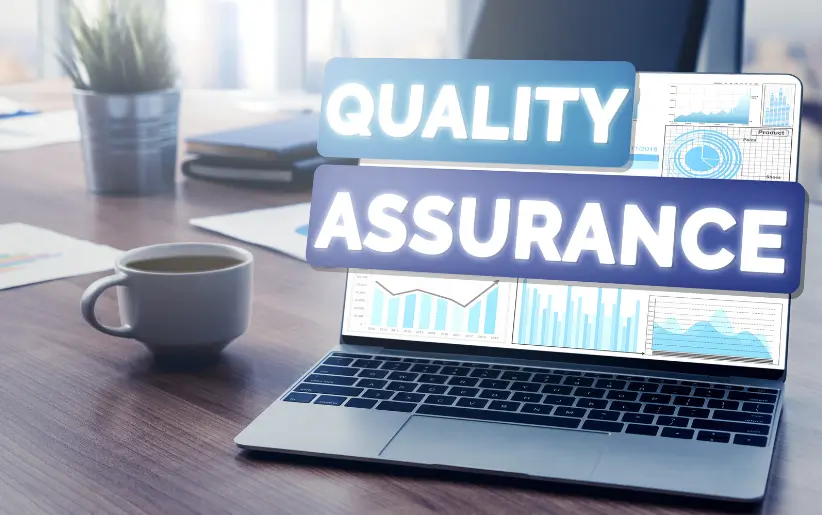
AI-enhanced workflow automation software represents a significant advancement in addressing the challenge of data quality. By leveraging advanced technologies such as AI-driven data cleansing, validation, and predictive analytics, AI can provide a comprehensive and efficient solution to ensure high-quality data. These systems offer organizations unprecedented levels of accuracy, reliability, and efficiency, ensuring that automated workflows operate seamlessly and effectively.
As technology continues to evolve, investing in AI-driven data quality solutions will become increasingly important for ensuring comprehensive workflow automation. Addressing challenges such as accuracy, data privacy, and cost will be crucial to fully realizing the potential of AI in workflow automation. Ultimately, AI represents a transformative force in the realm of workflow automation, offering innovative solutions that enhance data quality, efficiency, and business outcomes.
For further insights into AI and data quality in workflow automation, refer to McKinsey & Company’s report on AI-driven data integration and Gartner’s study on the cost of poor data quality.