“Innovation is the ability to see change as an opportunity – not a threat.”
Steve Jobs
AI-enhanced workflow automation software has redefined business operations, bringing unprecedented efficiency and productivity. Despite these advantages, a significant challenge that organizations encounter is interoperability. The ability of workflow automation systems to seamlessly integrate and operate with diverse software and hardware environments is critical. This article explores how AI-driven workflow automation software addresses the interoperability challenge, supported by comprehensive factual data, real-world applications, and an analysis of the broader implications for businesses.
The Complexity of Interoperability
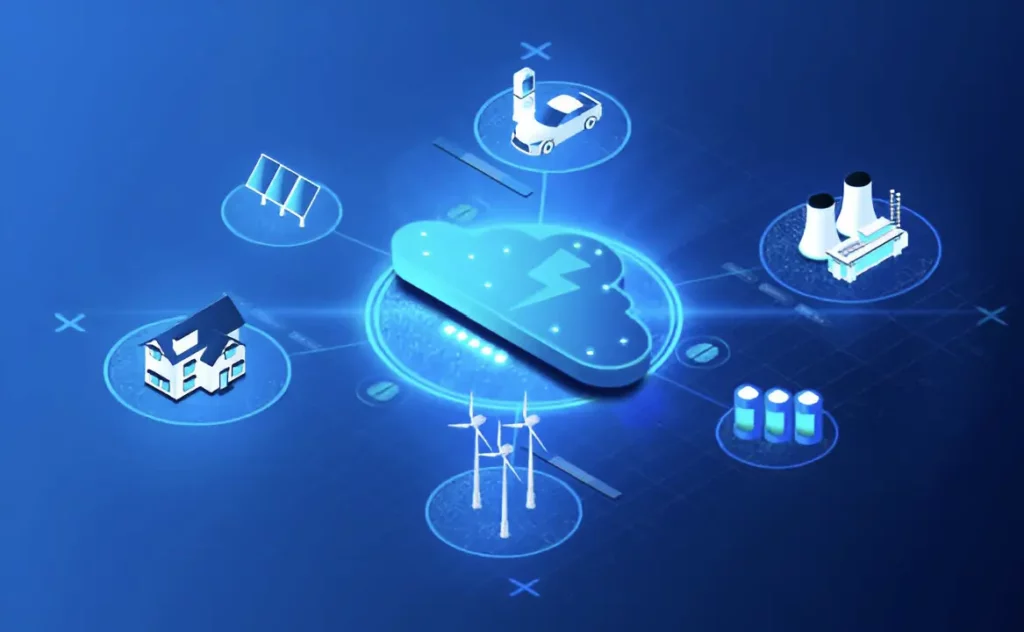
Interoperability refers to the ability of different systems and applications to work together seamlessly. For workflow automation to be effective, it must be able to integrate with a wide range of existing systems, including legacy applications, modern cloud services, and various third-party tools. However, achieving this integration is often complex due to the diverse nature of enterprise IT environments.
A report by IDC indicates that 60% of organizations face challenges related to system interoperability, which can lead to inefficiencies and increased operational costs. This complexity arises from differences in data formats, communication protocols, and system architectures. For example, an organization may use a mix of on-premises ERP systems, cloud-based CRM platforms, and various third-party applications. Ensuring that these systems can communicate and exchange data seamlessly is essential for effective workflow automation.
AI Solutions for Enhancing Interoperability
Artificial Intelligence offers powerful tools for addressing interoperability challenges in workflow automation. One significant advancement is the use of AI-driven integration platforms. These platforms leverage AI algorithms to facilitate seamless communication and data exchange between disparate systems. By automating the integration process, AI reduces the time and effort required for manual system integration, ensuring that workflows operate smoothly across diverse environments.
For instance, AI can analyze the data formats and communication protocols of different systems, identifying the best methods for integration. This analysis includes mapping data fields between systems, transforming data into compatible formats, and establishing secure communication channels. A study by Deloitte highlights that AI-driven integration can reduce integration costs by up to 30%, demonstrating the efficiency gains achieved through automation.
Another application of AI in enhancing interoperability is the use of machine learning for adaptive integration. Machine learning algorithms can continuously learn from system interactions and adapt integration processes to accommodate changes in system configurations and data structures. This capability ensures that the integration remains robust and flexible, even as the IT environment evolves. According to a report by Forrester, adaptive integration can improve system compatibility and reduce integration errors by up to 40%.
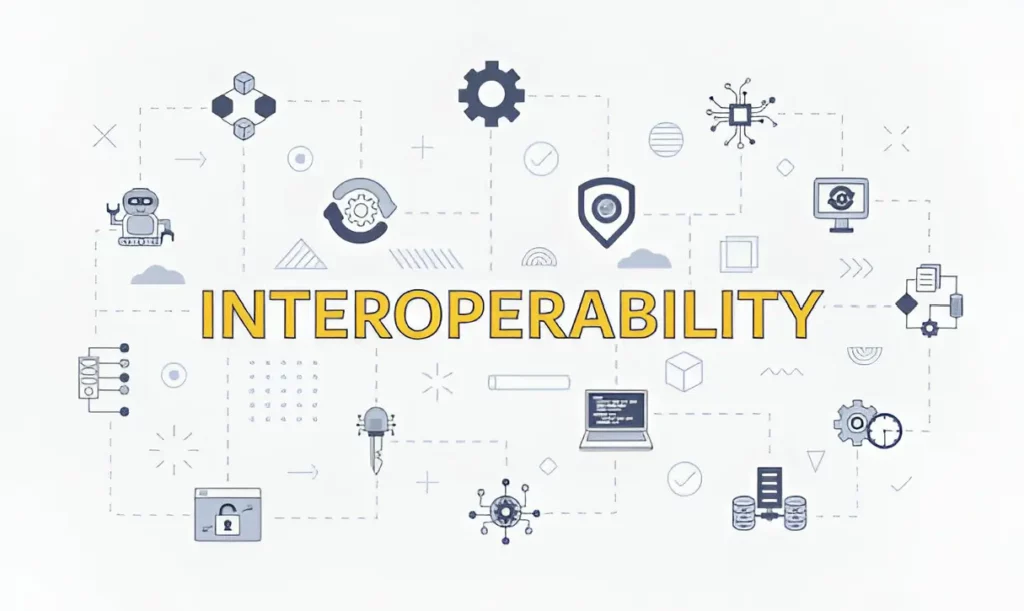
AI also enhances interoperability through the use of natural language processing (NLP) and intelligent APIs. NLP enables systems to understand and interpret human language, facilitating communication between systems that use different terminologies and data formats. Intelligent APIs provide a standardized interface for system integration, allowing different applications to communicate and exchange data seamlessly. These AI-driven capabilities ensure that workflow automation systems can operate effectively in diverse and complex IT environments.
Real-World Applications and Benefits
The practical application of AI in addressing interoperability challenges is evident in various innovative workflow automation platforms. Companies like MuleSoft and Dell Boomi have developed AI-powered solutions that prioritize interoperability and seamless integration.
MuleSoft’s Anypoint Platform uses AI-driven integration capabilities to connect disparate systems and applications. The platform’s AI algorithms analyze data formats and communication protocols, automating the integration process and ensuring seamless data exchange. MuleSoft’s Anypoint Platform also leverages machine learning for adaptive integration, continuously learning from system interactions and adjusting integration processes to accommodate changes in the IT environment.
Dell Boomi’s AtomSphere platform is another example of an AI-enhanced integration solution that addresses interoperability challenges. Dell Boomi’s platform uses AI-driven data mapping and transformation to facilitate seamless integration between different systems. The platform’s intelligent APIs provide a standardized interface for system communication, ensuring that applications can exchange data seamlessly. Additionally, Dell Boomi’s platform leverages NLP to interpret and translate different data formats, ensuring that workflow automation systems can operate effectively across diverse environments.
Addressing Challenges and Considerations
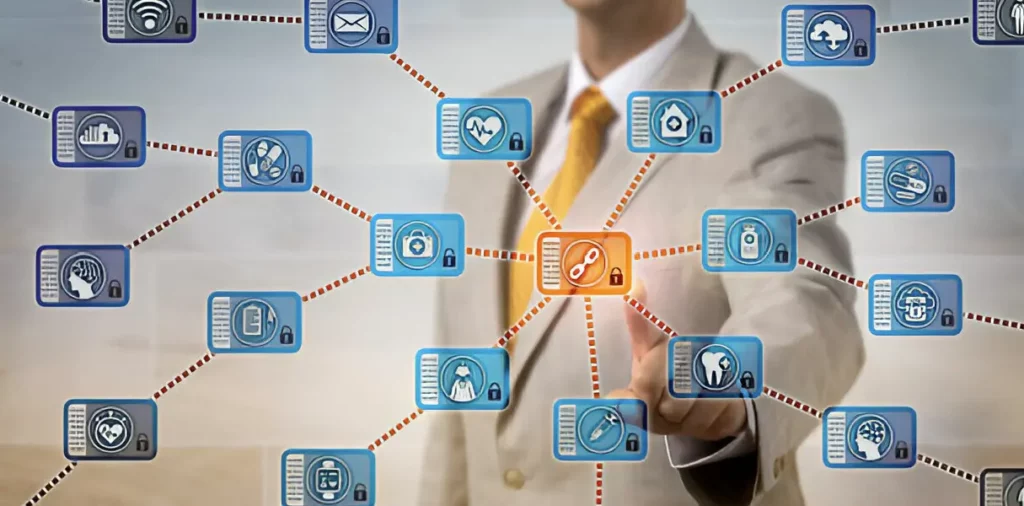
While AI offers significant benefits in enhancing interoperability, several challenges and considerations must be addressed. One primary concern is ensuring that AI-driven integration solutions are secure and reliable. Organizations must ensure that their AI systems are robust and resilient to handle complex integration scenarios without compromising security. Continuous monitoring and testing of AI algorithms are essential to maintain reliability and performance.
Data privacy and security are also critical considerations. AI-driven integration solutions process large volumes of sensitive data, making robust encryption and data protection measures essential. Transparency regarding data collection, storage, and usage policies is crucial to address privacy concerns and build confidence in AI-driven integration solutions.
The cost of AI-enabled integration solutions can also be a barrier to adoption. High-quality AI systems that provide advanced integration, machine learning, and NLP capabilities can be expensive. Ensuring that these systems are affordable and accessible is crucial for broader adoption and enhanced interoperability. Organizations must work together with technology providers to develop cost-effective solutions that do not compromise on quality and effectiveness.
Conclusion
AI-enhanced workflow automation software represents a significant advancement in addressing the challenge of interoperability. By leveraging advanced technologies such as AI-driven integration, machine learning, and natural language processing, AI can provide a comprehensive and efficient solution to ensure seamless interoperability. These systems offer organizations unprecedented levels of flexibility, efficiency, and reliability, ensuring that automated workflows can operate effectively across diverse IT environments.
As technology continues to evolve, investing in AI-driven interoperability solutions will become increasingly important for ensuring comprehensive workflow automation. Addressing challenges such as security, data privacy, and cost will be crucial to fully realizing the potential of AI in workflow automation. Ultimately, AI represents a transformative force in the realm of workflow automation, offering innovative solutions that enhance interoperability, efficiency, and business outcomes.
For further insights into AI and interoperability in workflow automation, refer to Deloitte’s report on AI-driven integration and Forrester’s study on adaptive integration.