“In God we trust; all others must bring data.”
W. Edwards Deming
The rise of AI-enhanced customer data platforms (CDPs) has transformed how businesses manage and utilize customer information, providing deep insights and driving more personalized marketing strategies. However, one significant challenge that persists is data fragmentation. As businesses collect data from multiple channels and touchpoints, ensuring that this information is consolidated and harmonized into a single, actionable view is critical. This article explores how AI-driven CDPs address the issue of data fragmentation, supported by comprehensive factual data, real-world applications, and an analysis of broader implications for businesses.
The Complexity of Data Fragmentation
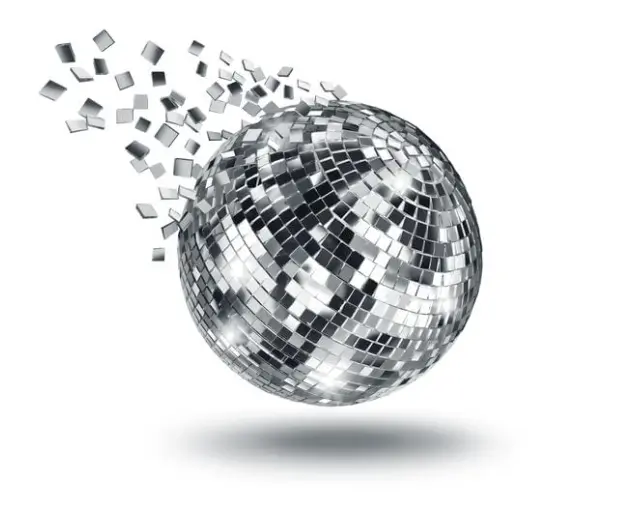
Data fragmentation occurs when customer information is dispersed across various systems and platforms, creating silos that hinder a comprehensive understanding of customer behavior. This fragmentation can lead to inconsistent and incomplete customer profiles, reducing the effectiveness of marketing strategies and customer engagement efforts. According to a study by Forrester, 57% of marketers say that integrating customer data from different sources is a significant challenge, highlighting the prevalence and impact of data fragmentation.
In today’s digital age, businesses collect data from a multitude of channels, including websites, social media, mobile apps, in-store interactions, and customer service engagements. Each of these channels generates valuable data that, when combined, can provide a holistic view of the customer. However, the sheer volume and variety of data sources often result in fragmented data, making it difficult to consolidate and analyze effectively.
AI Solutions for Enhancing Data Integration and Consolidation
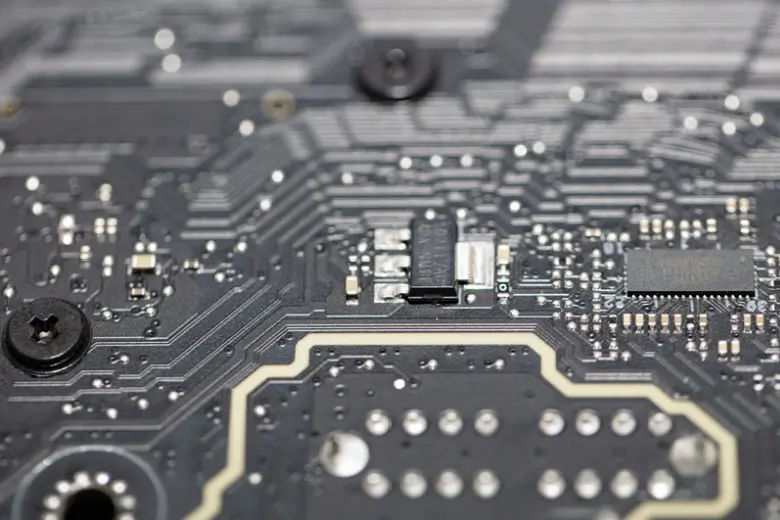
Artificial Intelligence offers powerful tools for addressing data fragmentation in customer data platforms. One significant advancement is the use of AI-driven data integration and consolidation. AI algorithms can automatically merge data from disparate sources, ensuring that it is harmonized and consistent. This automated process reduces the time and effort required for manual data integration, enhancing the overall quality and usability of customer data.
For instance, AI can analyze data from various sources, identify common data points, and merge them into a unified customer profile. This process involves matching and linking records based on identifiers such as email addresses, phone numbers, or customer IDs. A report by McKinsey & Company highlights that AI-driven data integration can improve data quality and completeness by up to 30%, demonstrating the efficiency gains achieved through automation.
Another application of AI in enhancing data consolidation is the use of machine learning for data deduplication and enrichment. Machine learning algorithms can identify and eliminate duplicate records, ensuring that each customer has a single, accurate profile. Additionally, AI can enrich customer profiles by filling in missing information using predictive analytics. For example, if a customer’s purchase history is incomplete, AI can predict likely purchases based on similar customer behaviors, providing a more comprehensive view of the customer.
AI also enhances data integration through natural language processing (NLP) and entity resolution. NLP enables the system to understand and interpret data from unstructured sources, such as customer service interactions or social media posts. Entity resolution techniques can identify and link related data points across different systems, ensuring that all relevant information is consolidated into a single customer profile. These AI-driven capabilities ensure that customer data platforms can provide a complete and accurate view of the customer, enhancing marketing and engagement strategies.
Real-World Applications and Benefits
The practical application of AI in addressing data fragmentation challenges is evident in various innovative customer data platforms. Companies like Salesforce and Segment have developed AI-powered solutions that prioritize data integration and consolidation.
Salesforce’s Customer 360 platform uses AI-driven data integration to merge data from various sources into a unified customer profile. The platform’s AI algorithms analyze data from different channels, identify common data points, and consolidate them into a single view. Salesforce Customer 360 also leverages machine learning for data deduplication and enrichment, ensuring that customer profiles are accurate and comprehensive. Additionally, Salesforce’s NLP capabilities enable the platform to interpret data from unstructured sources, enhancing the overall quality and completeness of customer data.
Segment’s CDP is another example of an AI-enhanced solution that addresses data fragmentation challenges. Segment’s platform uses AI-driven entity resolution to link related data points across different systems, ensuring that all relevant information is consolidated into a single customer profile. The platform’s machine learning algorithms identify and eliminate duplicate records, providing a single, accurate view of the customer. Segment’s CDP also leverages NLP to interpret data from unstructured sources, ensuring that customer profiles are complete and comprehensive.
Navigating the Challenges and Considerations
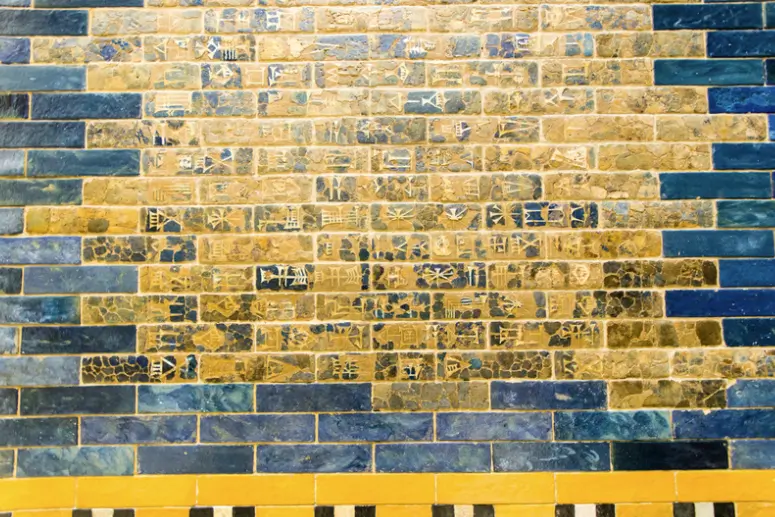
While AI offers significant benefits in enhancing data integration and consolidation, several challenges and considerations must be addressed. One primary concern is ensuring that AI-driven data integration solutions are accurate and reliable. Organizations must ensure that their AI systems are trained on comprehensive and up-to-date data to avoid errors and omissions. Continuous validation and testing of AI algorithms are essential to maintaining accuracy and reliability.
Data privacy and security are also critical considerations. AI-driven data integration solutions process large volumes of sensitive customer information, making robust encryption and data protection measures essential. Transparency regarding data collection, storage, and usage policies is crucial to address privacy concerns and build confidence in AI-driven data integration solutions.
The cost of AI-enabled data integration solutions can also be a barrier to adoption. High-quality AI systems that provide advanced data integration, machine learning, and NLP capabilities can be expensive. Ensuring that these systems are affordable and accessible is crucial for broader adoption and enhanced data integration. Organizations must work together with technology providers to develop cost-effective solutions that do not compromise on quality and effectiveness.
Conclusion
AI-enhanced customer data platforms represent a significant advancement in addressing the challenge of data fragmentation. By leveraging advanced technologies such as AI-driven data integration, machine learning, and natural language processing, AI can provide a comprehensive and efficient solution to ensure seamless data consolidation. These systems offer organizations unprecedented levels of data quality, completeness, and usability, ensuring that customer data platforms provide a complete and accurate view of the customer.
As technology continues to evolve, investing in AI-driven data integration solutions will become increasingly important for ensuring comprehensive customer data management. Addressing challenges such as accuracy, data privacy, and cost will be crucial to fully realizing the potential of AI in customer data platforms. Ultimately, AI represents a transformative force in the realm of customer data management, offering innovative solutions that enhance data integration, quality, and business outcomes.
For further insights into AI and data integration in customer data platforms, refer to McKinsey & Company’s report on AI-driven data integration and Forrester’s study on data integration challenges.