“The goal is to turn data into information, and information into insight.”
Carly Fiorina
The advent of AI-enhanced customer data platforms (CDPs) has revolutionized how businesses handle and leverage customer data, driving more personalized and effective marketing strategies. Yet, one of the significant challenges these platforms face is real-time data processing. As businesses strive to provide timely and relevant customer experiences, the ability to process and analyze data in real-time becomes crucial. This article explores how AI-driven CDPs address the challenge of real-time data processing, supported by comprehensive factual data, real-world applications, and a thorough analysis of broader implications for businesses.
The Criticality of Real-Time Data Processing
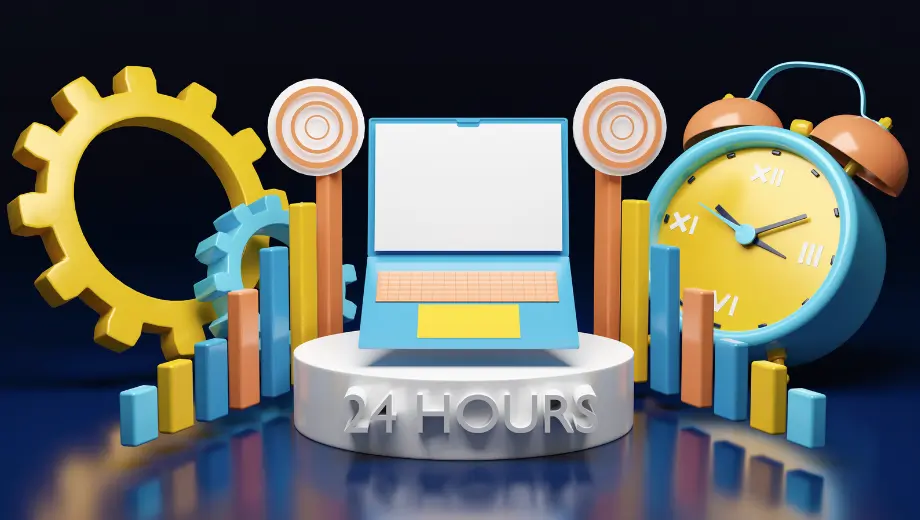
Real-time data processing is essential for delivering immediate and relevant customer experiences. In an era where customers expect instant responses and personalized interactions, businesses must process and analyze customer data as it is generated. This capability allows organizations to engage with customers in the moment, providing timely offers, recommendations, and support. However, achieving real-time data processing is a complex task that requires sophisticated technology and infrastructure.
According to a report by IDC, 70% of enterprises recognize the importance of real-time data processing for enhancing customer experiences, yet only 30% have implemented real-time data solutions. This disparity highlights the challenges that organizations face in adopting real-time data processing technologies. These challenges include the need for high-speed data integration, real-time analytics, and seamless data flow across various systems.
AI Solutions for Real-Time Data Processing
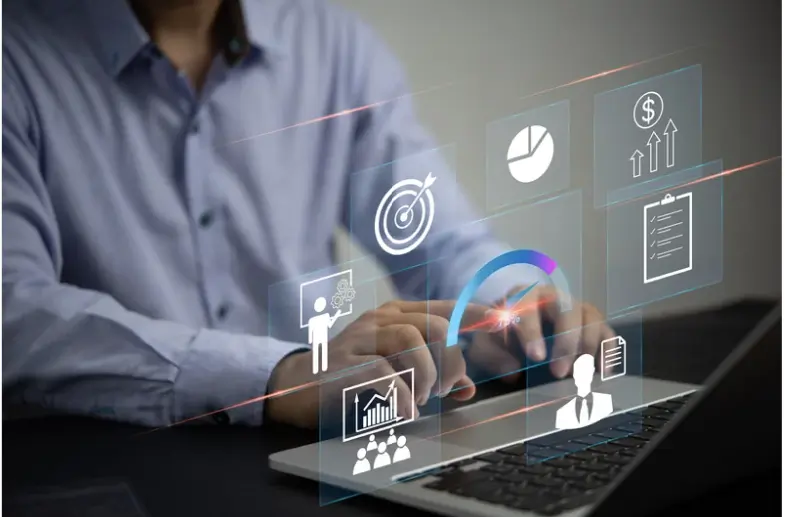
Artificial Intelligence offers robust solutions for overcoming the challenges of real-time data processing in customer data platforms. One significant advancement is the use of AI-driven stream processing. AI algorithms can process and analyze data streams in real-time, enabling businesses to gain immediate insights and take swift action. This capability is crucial for responding to customer interactions as they occur, ensuring that engagement is timely and relevant.
For example, AI can analyze customer data streams from various sources, such as website interactions, social media activity, and transactional data, to identify trends and patterns in real-time. This analysis allows businesses to detect customer intent and preferences, enabling them to deliver personalized offers and recommendations instantly. A study by McKinsey & Company highlights that real-time analytics can increase customer satisfaction by up to 20%, demonstrating the impact of timely engagement on customer experiences.
Another application of AI in enhancing real-time data processing is the use of machine learning for predictive analytics. Machine learning algorithms can analyze historical data to predict future customer behaviors and preferences. These predictions can be generated in real-time, allowing businesses to anticipate customer needs and take proactive measures. According to a report by Forrester, predictive analytics can improve marketing ROI by up to 15%, highlighting the value of real-time insights in driving business outcomes.
AI also enhances real-time data processing through natural language processing (NLP) and intelligent automation. NLP enables the system to interpret and analyze unstructured data from sources such as customer reviews, social media posts, and support tickets in real-time. Intelligent automation ensures that these insights are seamlessly integrated into customer engagement strategies, enabling businesses to act on real-time data without delay. These AI-driven capabilities ensure that customer data platforms can provide timely and relevant insights, enhancing customer experiences and business performance.
Real-World Applications and Benefits
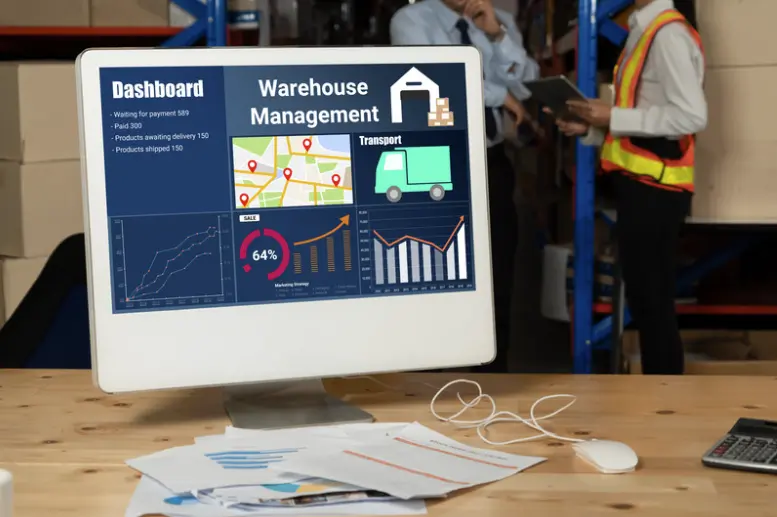
The practical application of AI in addressing real-time data processing challenges is evident in various innovative customer data platforms. Companies like Snowflake and Adobe have developed AI-powered solutions that prioritize real-time data processing and analytics.
Snowflake’s Data Cloud platform leverages AI-driven stream processing to analyze data in real-time. The platform’s AI algorithms process data streams from various sources, providing immediate insights and enabling timely customer engagement. Snowflake’s machine learning capabilities also facilitate predictive analytics, allowing businesses to anticipate customer needs and deliver personalized experiences proactively.
Adobe’s Experience Platform is another example of an AI-enhanced customer data platform that addresses real-time data processing challenges. Adobe’s platform uses AI-driven stream processing to analyze customer data in real-time, providing immediate insights and enabling timely engagement. The platform’s NLP capabilities allow it to interpret unstructured data from various sources, ensuring that insights are comprehensive and actionable. Adobe’s intelligent automation ensures that these insights are seamlessly integrated into customer engagement strategies, enhancing the overall customer experience.
Navigating the Challenges and Considerations
While AI offers significant benefits in enhancing real-time data processing, several challenges and considerations must be addressed. One primary concern is ensuring that AI-driven real-time data processing solutions are scalable and resilient. Organizations must ensure that their AI systems can handle high volumes of data and maintain performance under varying conditions. Continuous monitoring and optimization of AI algorithms are essential to maintaining scalability and reliability.
Data privacy and security are also critical considerations. AI-driven real-time data processing involves handling large volumes of sensitive customer information, making robust encryption and data protection measures essential. Transparency regarding data collection, storage, and usage policies is crucial to address privacy concerns and build confidence in AI-driven real-time data processing solutions.
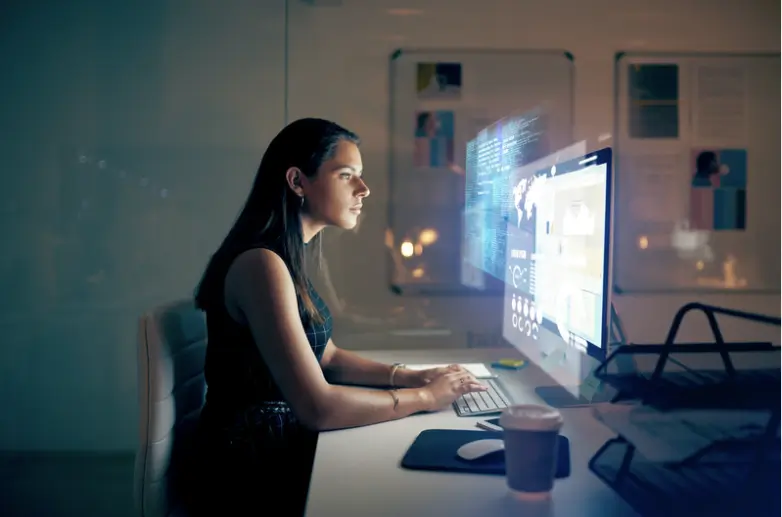
The cost of AI-enabled real-time data processing solutions can also be a barrier to adoption. High-quality AI systems that provide advanced stream processing, predictive analytics, and intelligent automation capabilities can be expensive. Ensuring that these systems are affordable and accessible is crucial for broader adoption and enhanced real-time data processing. Organizations must work together with technology providers to develop cost-effective solutions that do not compromise on quality and effectiveness.
Conclusion
AI-enhanced customer data platforms represent a significant advancement in addressing the challenge of real-time data processing. By leveraging advanced technologies such as AI-driven stream processing, machine learning, and natural language processing, AI can provide a comprehensive and efficient solution to ensure timely and relevant customer engagement. These systems offer organizations unprecedented levels of data speed, accuracy, and usability, ensuring that customer data platforms provide real-time insights and enhance customer experiences.
As technology continues to evolve, investing in AI-driven real-time data processing solutions will become increasingly important for ensuring comprehensive customer data management. Addressing challenges such as scalability, data privacy, and cost will be crucial to fully realizing the potential of AI in customer data platforms. Ultimately, AI represents a transformative force in the realm of customer data management, offering innovative solutions that enhance real-time data processing, customer engagement, and business outcomes.
For further insights into AI and real-time data processing in customer data platforms, refer to McKinsey & Company’s report on real-time analytics and Forrester’s study on predictive analytics.