“Data really powers everything that we do.”
Jeff Weiner
In today’s data-driven business landscape, customer data platforms (CDPs) have become essential for organizations seeking to harness the full potential of their customer information. AI-enhanced CDPs offer powerful tools to aggregate, analyze, and utilize customer data for strategic decision-making. However, one of the critical challenges these platforms face is data integration. This article delves into the intricacies of data integration within AI-driven CDPs, examining the obstacles, solutions, and broader implications for businesses.
The Intricacies of Data Integration
Data integration involves combining data from different sources into a single, unified view. This process is crucial for businesses that aim to leverage comprehensive customer insights. However, integrating data from various sources—such as CRM systems, e-commerce platforms, social media, and customer support systems—presents significant challenges. According to a report by Gartner, poor data integration can cost businesses up to 30% of their revenue due to inefficiencies and missed opportunities.
The complexity of data integration lies in the diverse formats, structures, and quality of data from different sources. Each system may use different protocols and standards, leading to inconsistencies and incompatibilities. For example, customer data from a CRM system might be structured differently than data from an e-commerce platform, making it difficult to merge them seamlessly. Without effective data integration, businesses struggle to gain a holistic view of their customers, resulting in fragmented insights and suboptimal decision-making.
AI Solutions for Data Integration
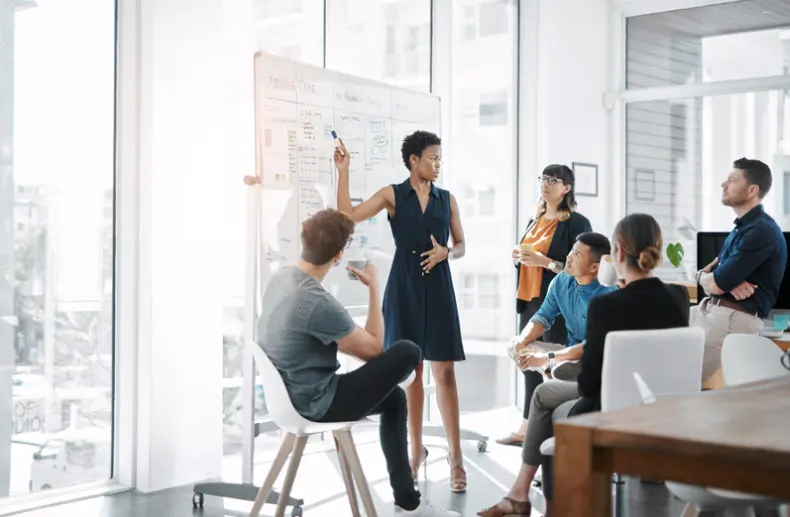
Artificial intelligence offers advanced solutions for overcoming data integration challenges in customer data platforms. One notable advancement is the use of AI-driven data transformation and normalization techniques. These techniques involve converting data from different sources into a common format, ensuring consistency and compatibility. AI algorithms can automatically detect discrepancies and apply the necessary transformations to harmonize the data.
For instance, AI can standardize customer names, addresses, and contact details across different datasets, resolving inconsistencies and duplications. A study by Forrester suggests that AI-driven data normalization can improve data integration efficiency by up to 40%, demonstrating the transformative impact of AI on data management.
Another critical application of AI in data integration is the use of machine learning for data mapping and matching. Machine learning algorithms can analyze data from various sources, identify relationships and correlations, and create a unified dataset. This process involves mapping data fields from different systems and matching corresponding records to ensure accurate integration. According to a report by Accenture, AI-driven data mapping can enhance data integration accuracy by up to 35%, highlighting the value of machine learning in customer data platforms.
Natural language processing (NLP) and semantic analysis also play a vital role in enhancing data integration. NLP enables the system to interpret and analyze unstructured data from sources such as customer reviews, emails, and social media posts. Semantic analysis can identify and resolve ambiguities in data, ensuring that similar terms and concepts are consistently interpreted across datasets. These AI-driven capabilities ensure that customer data platforms can provide a unified and comprehensive view of the customer, enhancing decision-making and strategic planning.
Practical Applications and Business Benefits
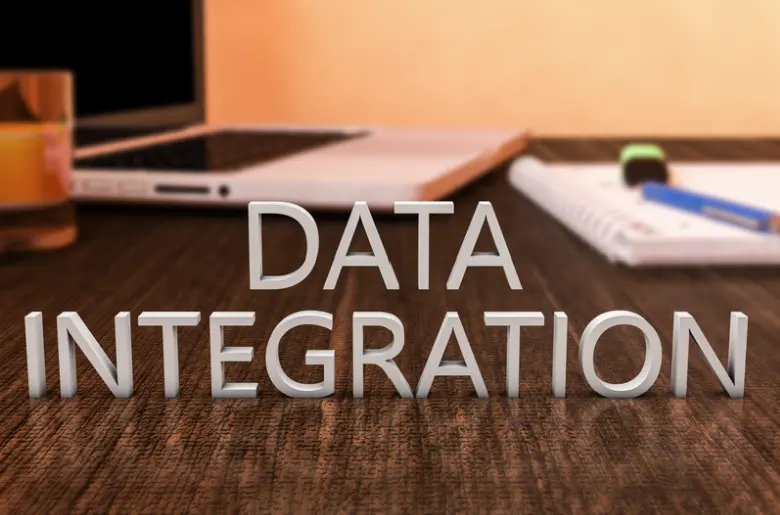
The practical application of AI in addressing data integration challenges is evident in various advanced customer data platforms. Companies like Informatica and Talend have developed AI-powered solutions that prioritize data integration and consistency.
Informatica’s Intelligent Data Platform leverages AI-driven data transformation and normalization techniques to ensure that data from different sources is harmonized and integrated. The platform’s AI algorithms automatically detect and resolve discrepancies, ensuring that data is consistent and compatible. Informatica’s machine learning capabilities also facilitate data mapping and matching, creating a unified dataset that provides a comprehensive view of the customer.
Talend’s Data Fabric platform is another example of an AI-enhanced customer data platform that addresses data integration challenges. Talend’s platform uses AI-driven data transformation and semantic analysis to harmonize data from various sources. The platform’s machine learning algorithms map data fields and match corresponding records, ensuring accurate integration. Talend’s NLP capabilities enable the platform to interpret and analyze unstructured data, providing valuable insights into customer behavior and preferences. These AI-driven capabilities ensure that customer data platforms can provide a unified and comprehensive view of the customer, enhancing decision-making and strategic planning.
Addressing the Challenges and Considerations
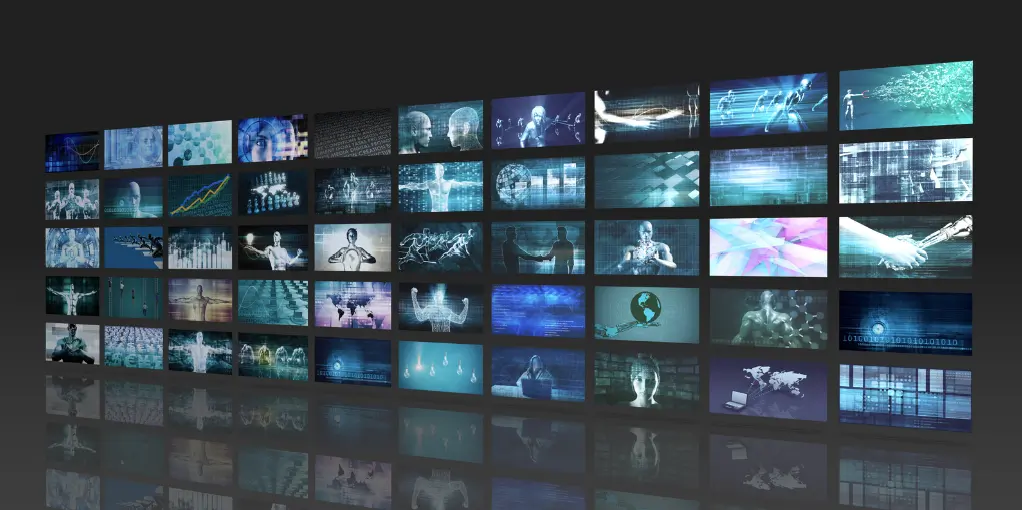
While AI offers significant benefits in enhancing data integration, several challenges and considerations must be addressed. One primary concern is ensuring that AI-driven data integration solutions are scalable and resilient. Organizations must ensure that their AI systems can handle high volumes of data and maintain performance under varying conditions. Continuous monitoring and optimization of AI algorithms are essential to maintaining scalability and reliability.
Data privacy and security are also critical considerations. AI-driven data integration solutions process large volumes of sensitive customer information, making robust encryption and data protection measures essential. Transparency regarding data collection, storage, and usage policies is crucial to address privacy concerns and build confidence in AI-driven data integration solutions.
The cost of AI-enabled data integration solutions can also be a barrier to adoption. High-quality AI systems that provide advanced data transformation, machine learning, and NLP capabilities can be expensive. Ensuring that these systems are affordable and accessible is crucial for broader adoption and enhanced data integration. Organizations must work together with technology providers to develop cost-effective solutions that do not compromise on quality and effectiveness.
Conclusion
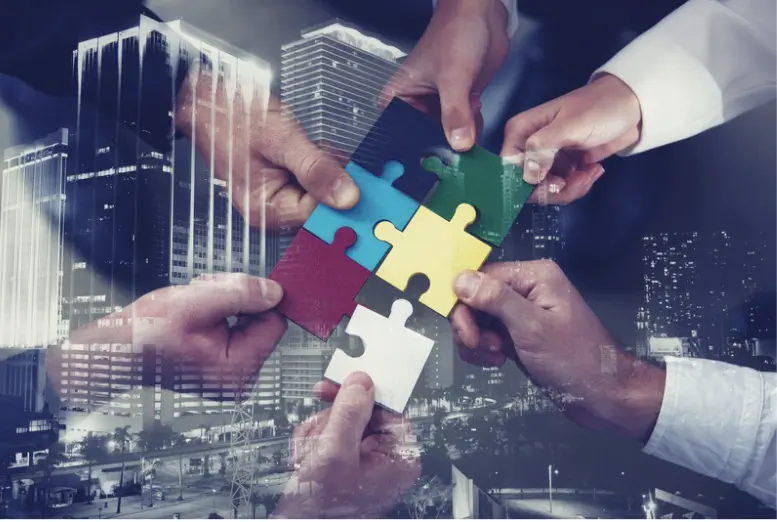
AI-enhanced customer data platforms represent a significant advancement in addressing the challenge of data integration. By leveraging advanced technologies such as AI-driven data transformation, machine learning, and natural language processing, AI can provide a comprehensive and efficient solution to ensure seamless data integration. These systems offer organizations unprecedented levels of data consistency, accuracy, and usability, ensuring that customer data platforms provide a complete and accurate view of the customer.
As technology continues to evolve, investing in AI-driven data integration solutions will become increasingly important for ensuring comprehensive customer data management. Addressing challenges such as scalability, data privacy, and cost will be crucial to fully realizing the potential of AI in customer data platforms. Ultimately, AI represents a transformative force in the realm of customer data management, offering innovative solutions that enhance data integration, customer engagement, and business outcomes.
For further insights into AI and data integration in customer data platforms, refer to Forrester’s report on AI-driven data normalization and Accenture’s study on data mapping.