Information is the oil of the 21st century, and analytics is the combustion engine.”
Peter Sondergaard
In an increasingly data-driven world, customer data platforms (CDPs) have become indispensable tools for businesses seeking to harness customer information for strategic insights and personalized marketing. However, as these platforms accumulate vast amounts of data, the issue of data redundancy becomes a significant challenge. This article explores the intricacies of data redundancy in AI-driven CDPs, examining the underlying problems, AI-powered solutions, and broader implications for businesses.
Understanding Data Redundancy in CDPs
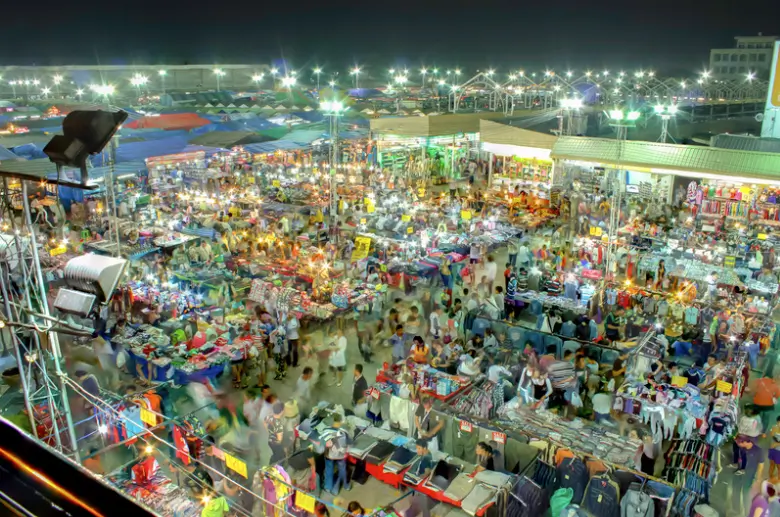
Data redundancy occurs when the same piece of data is stored in multiple places within a database or across systems. While some redundancy is intentional and can provide data backup and fault tolerance, excessive redundancy leads to inefficiencies and increased storage costs. In the context of CDPs, data redundancy can result from the integration of multiple data sources, where overlapping data from CRM systems, social media, e-commerce platforms, and other touchpoints is stored without proper consolidation.
The consequences of data redundancy in CDPs include inconsistent data, inflated storage requirements, and reduced system performance. According to a report by IDC, businesses waste an estimated $3.1 trillion annually due to poor data management practices, including redundancy. Redundant data can lead to inaccurate analytics, misinformed decision-making, and ultimately, suboptimal customer engagement strategies.
AI Solutions for Data Redundancy
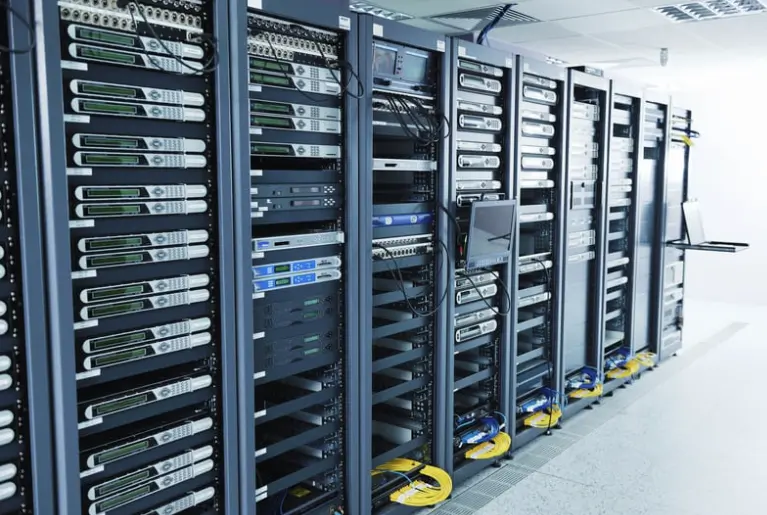
Artificial intelligence offers advanced tools to address data redundancy challenges in customer data platforms. One significant advancement is the use of AI for data deduplication. Data deduplication involves identifying and eliminating duplicate data entries to ensure that each piece of data is stored only once. AI algorithms can analyze vast datasets to detect duplicates based on various parameters such as customer names, email addresses, transaction details, and more.
Machine learning models can learn from existing data patterns to improve the accuracy of deduplication processes over time. For instance, AI can identify slight variations in customer data—such as misspellings or different formats—that might otherwise be overlooked. By consolidating these variations into single, accurate records, AI-driven deduplication can significantly enhance data quality. According to a study by Gartner, implementing AI-driven data deduplication can reduce storage requirements by up to 70%, demonstrating its potential to streamline data management.
Another critical application of AI in addressing data redundancy is the use of natural language processing (NLP) to consolidate unstructured data. NLP can analyze and interpret text data from customer reviews, emails, and social media posts, identifying similarities and consolidating redundant information. This capability ensures that all relevant customer data is included in the integrated dataset without unnecessary duplication.
Practical Applications and Business Benefits
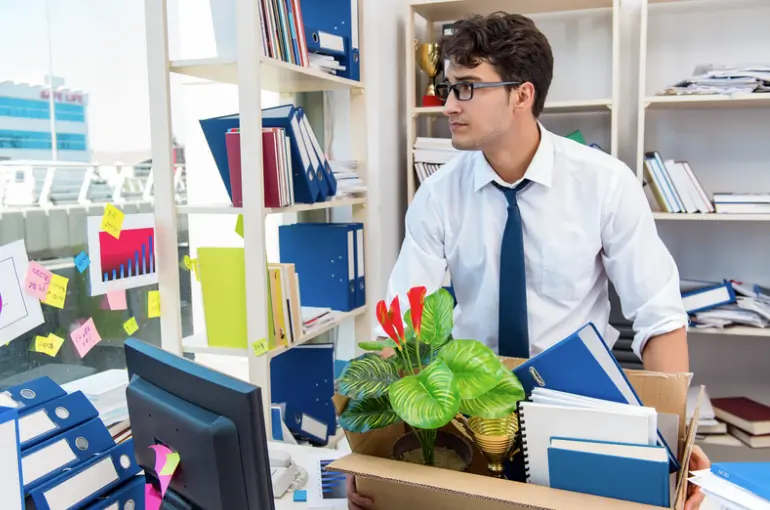
Several advanced customer data platforms leverage AI to address data redundancy challenges. For instance, Informatica’s Intelligent Cloud Services use AI-driven data deduplication to ensure that customer data is accurate and comprehensive. The platform’s machine learning algorithms automatically detect and eliminate duplicate records, enhancing data quality and reducing storage costs.
Talend’s Data Fabric platform is another example of an AI-enhanced customer data platform that addresses data redundancy challenges. Talend’s AI algorithms facilitate the deduplication of data from different systems, ensuring consistency and accuracy. The platform’s NLP features enable it to integrate unstructured data sources, such as customer feedback and social media interactions, providing a complete view of customer behavior without redundancy.
Overcoming Data Redundancy Challenges
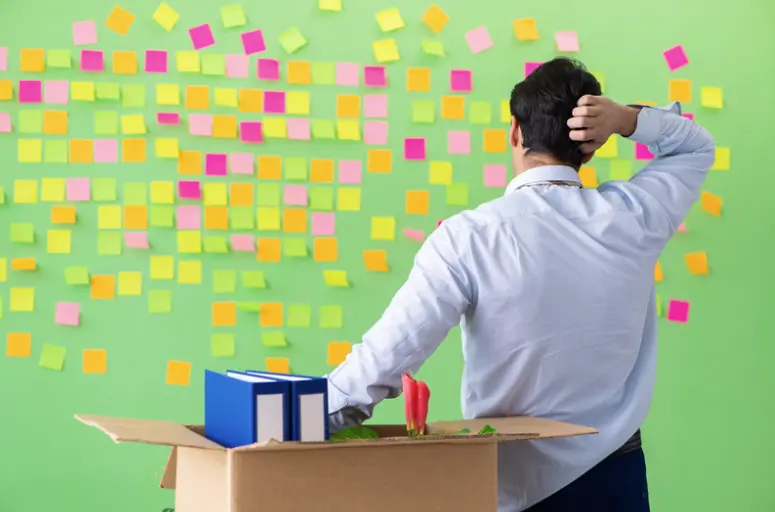
Despite the advancements in AI-driven data redundancy solutions, several challenges remain. One primary concern is ensuring the accuracy of deduplication processes. Businesses must ensure that their AI-driven deduplication solutions are accurate and reliable to avoid the inadvertent removal of important data. This accuracy is crucial for maintaining data integrity and ensuring the reliability of analytics.
Data privacy and security are also critical considerations. AI-driven data redundancy solutions process large volumes of sensitive customer information, making robust encryption and data protection measures essential. Ensuring compliance with data privacy regulations, such as GDPR and CCPA, is crucial to maintaining customer trust and avoiding legal penalties.
The cost of implementing AI-driven data redundancy solutions can also be a barrier for some businesses. High-quality AI systems that provide advanced deduplication and NLP capabilities can be expensive. However, the long-term benefits of enhanced data quality, reduced storage costs, and improved system performance often justify the initial investment.
Conclusion
AI-enhanced customer data platforms represent a significant advancement in addressing data redundancy challenges. By leveraging technologies such as AI-driven data deduplication and natural language processing, businesses can ensure robust data management while leveraging customer data for strategic insights. These systems offer unprecedented levels of data quality, consistency, and efficiency, ensuring that customer data platforms provide a reliable foundation for business operations.
As digital transformation continues to accelerate, investing in AI-driven data redundancy solutions will become increasingly important for businesses seeking to optimize their data management strategies. Addressing challenges such as accuracy, data privacy, and cost will be crucial to fully realizing the potential of AI in customer data platforms. Ultimately, AI represents a transformative force in the realm of data management, offering innovative solutions that enhance data quality, customer insights, and business outcomes.
For further insights into AI and data redundancy in customer data platforms, refer to Gartner’s report on AI-driven data deduplication and IDC’s study on data management inefficiencies.