In an industry where efficiency and reliability are paramount, artificial intelligence (AI) has emerged as a transformative force. Particularly in manufacturing, AI-driven predictive maintenance is reshaping how businesses approach machinery upkeep, system reliability, and downtime reduction. By integrating AI into their maintenance strategies, manufacturers can anticipate issues before they escalate into costly repairs or operational halts, ensuring continuous productivity and improved safety standards.
Understanding Predictive Maintenance in Manufacturing
Predictive maintenance refers to the technique of using data analysis tools and techniques to detect anomalies in the operation and potential failures of machines before they occur. Unlike traditional maintenance practices that follow set schedules or respond after failures, predictive maintenance anticipates problems, allowing for timely intervention. This approach leverages various forms of data from machines, including vibration analysis, temperature readings, and historical performance data, to predict equipment failures with significant accuracy.
The Role of AI in Enhancing Predictive Maintenance
AI enhances predictive maintenance by processing and analyzing large volumes of data in real-time, learning from this data to identify patterns or anomalies that precede equipment failures. AI algorithms can continuously improve their diagnostic accuracy through machine learning, adapting to new conditions without explicit reprogramming. This capability not only increases the reliability of predictions but also optimizes maintenance schedules, reducing unnecessary inspections and focusing resources on when and where they are truly needed.
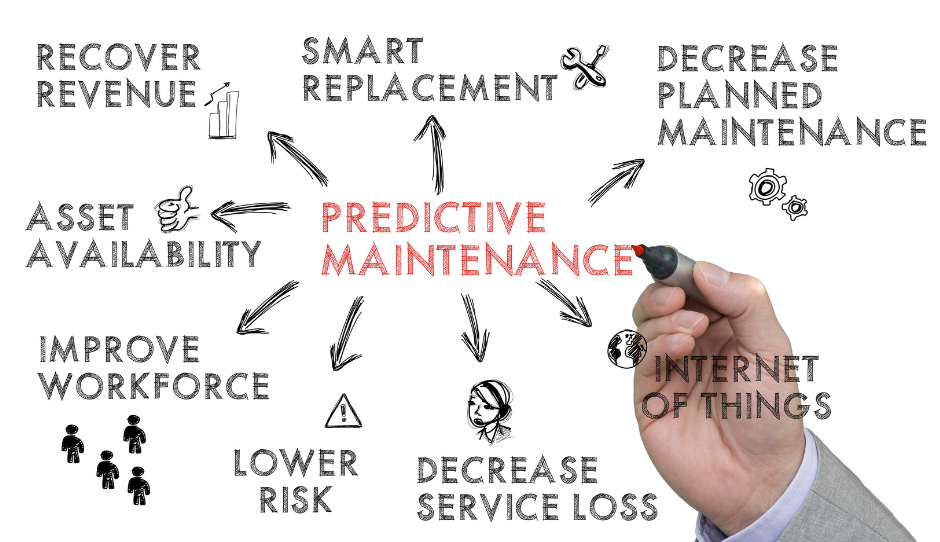
Detailed Monitoring and Analysis: AI systems employ advanced sensors and IoT (Internet of Things) technology to monitor equipment conditions continuously. These systems analyze data from sensors to detect subtle changes that may indicate wear or an impending breakdown. For instance, an increase in vibration or heat can suggest issues like misalignment or lubrication deficiencies that, if left unchecked, could lead to costly failures.
Automating Routine Checks: Through automation, AI systems can perform regular checks on machinery without human intervention, ensuring that no anomaly goes unnoticed, regardless of the human workforce’s limitations. This constant vigilance helps in maintaining high standards of operation and safety.
Enhancing Decision-Making: AI-driven predictive maintenance provides actionable insights that help plant managers make informed decisions about when maintenance should occur. It effectively prioritizes maintenance tasks based on the severity and risk associated with potential failures, ensuring that resources are allocated efficiently.
Implementing AI in Predictive Maintenance
Integration with Existing Systems: For successful implementation, AI technologies must be seamlessly integrated with existing manufacturing systems. This integration involves setting up sensors and data collection points on critical equipment and training the AI models with historical data to understand baseline operational behaviors.
Staff Training and Adaptation: Equipping staff with the necessary skills to work alongside AI tools is crucial. Training programs that focus on the operation and interpretation of AI-driven systems can empower employees, turning them into proactive participants in maintenance rather than reactive responders to breakdowns.
Continuous Improvement and Scaling: As AI systems gather more data over time, their predictions become more accurate and encompassing. Manufacturers can scale these systems across multiple facilities, applying lessons learned and data-driven insights across the entire enterprise.
The Future of Manufacturing with AI
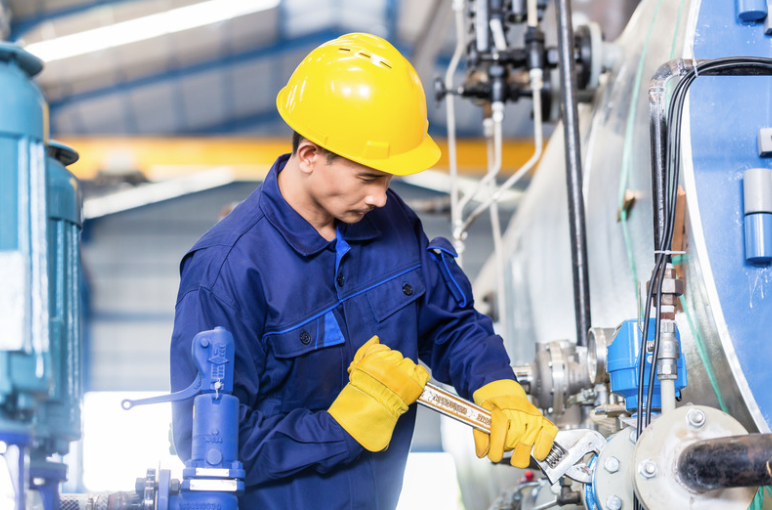
As AI technology continues to evolve, its integration into predictive maintenance strategies is expected to become more sophisticated. With advancements in AI capabilities, predictive maintenance will not only anticipate when a machine will fail but also recommend the most effective maintenance procedures and automate various repair processes.
PeakMet’s Contribution to AI-Driven Predictive Maintenance
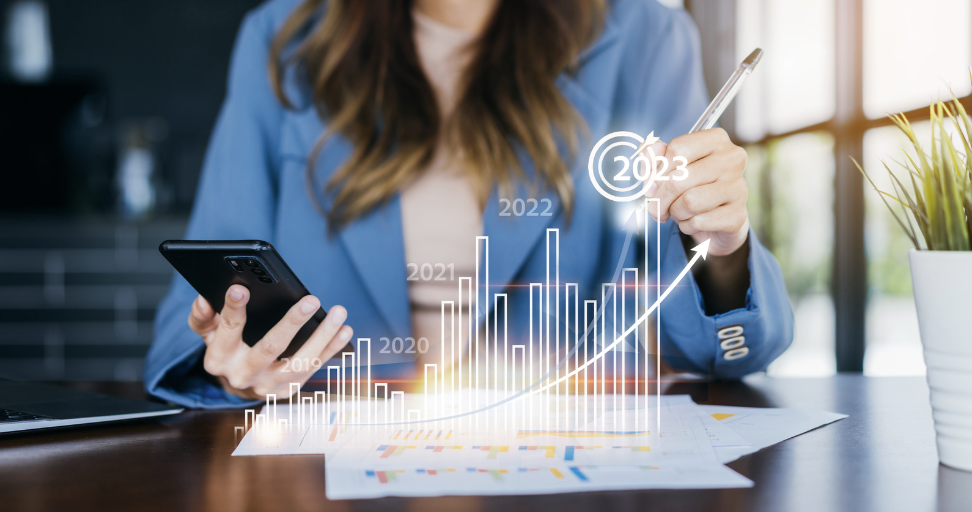
PeakMet provides a platform that enhances AI’s capabilities in predictive maintenance. By offering customized AI solutions that fit specific manufacturing needs, PeakMet helps businesses harness the full potential of their data. It provides tools for deeper data analysis, more accurate predictions, and effective decision-making that can revolutionize maintenance strategies.
In conclusion, AI-driven predictive maintenance in manufacturing represents a significant leap forward in how industries manage their operations. By predicting and preemptively addressing potential failures, manufacturers can reduce downtime, save on repair costs, and maintain a competitive edge in the market. With AI tools like PeakMet, the future of manufacturing looks not only more efficient but also smarter and more resilient.